Notes from Data2030 Conference, Stockholm
29 September 2022
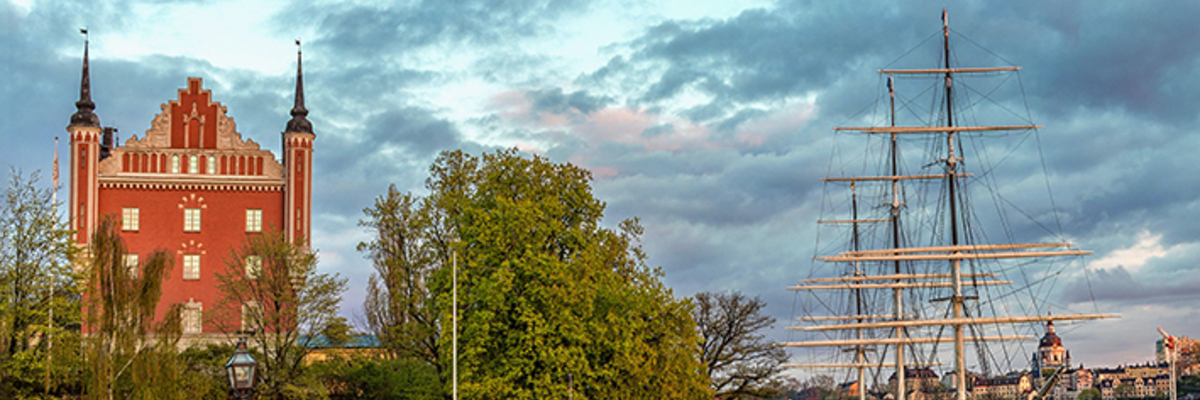
Data 2030, Stockholm
Data projects: a team event in extreme
Lotte Ansgaard Thomsen, Lead Data Architect at AI Solution Grundfos
70% of data projects tend to fail and even more so for pure AI projects. How can this be mitigated. Any project needs to start with a good "business case". A data project is iterative and circular. Data projects need to be multidisciplinary with data engineers and data scientists but also with business owners and domain experts. The callenge is that these people are often in different organizational units, allowing too often for escalations. Every hierarchical escalation stops the iterative process of the data project.
We can ask the question, "will data mesh help with this?" A multi-disciplinary team could be seen as a "data mesh node". Key takeaway is to organize for collaboration, spend ample time on defining the business idea, have an AI/analytics strategy, have knowledge of your end-to-end data flow. And get started sooner than later.
How to have and eat your cake - Solving Data Paradoxes in AI Training
Mats Nordlund, Head of Data Factory at AI.SE
We need to think about Sweden as a superpower. There is no other country (at this size) that has such a broad industrial base. AI Sweden accelerates the use of AI in companies and government. There are about 110 partners. There are no NDAs, no IP contracts, etc. That allows to compete for speed, instead of negotiating contracts.
AI Sweden created a data factory and a research arena, one focusses on the infrastructure, annotation, data and hardware. The other focusses on the ideas, the innovationn the interactions,... AI Sweden wants to be complementary to resources that already exist in Sweden and as such be part of the AI ecosystem, not build a second one. A great trick to speed is to have no money. Having no money leads to partners "giving" equipment, cutting out procurement processes and legal disputes.
The model of massive data hoarding is not sustainable. We want more data but we get less, we want to share data but need to keep it private, we want to exchange data but need to keep it local.
Data is born on the edge (e.g. in your car, hospital or phone). Can we not keep it there? Trends to look at is edge learning and swarm learning. AI Sweden created an Edge Learning Lab.
AI Sweden is like a gym. The more partners come to work out, the stronger you get faster.
The Edge learing consortium focusses on initial research areas (including space, healthcare,..), including cyber security.
Other labs are coming up, such as Language Lab, Energy Lab,...
Find out more at my.ai.se
Maximise the potential of your data to empower your future
Grag Hanson, Evangelist & VP EMEA & LATAM Informatica and Maria Ana Liz, Head of Data & Analytics Self Service Ericsson
Common challenges today are the following. Do you understand your data assets? If you don't, you will need to discover them. Can you access the data? You may need to move it to where you can access it. Is it of the appropriate quality? Do you have a single view of data? Can you ensure protection and privacy? And how do you offer data consumption in a governed way?
Large organizations are very much challenged by data complexity. 55% of organizations report that data quality is a top bottleneck, 75% of employees don't feel well prepared to use data effectively and compliantly. 37% of time in a data-driven activity is lost looking for data.
For Ericsson, having the top management support was a key success factor. Ericsson started by investing in new technology but quickly realized that the cultural shift to understand the technology and its potential was not there. Data strategy should be a business strategy, coming from a value approach, not a compliance approach, nor a technology approach. "Making decisions on bad data is making bad decisions faster than before." The investment in the "data market place" was most likely the most impactful one. The marketplace was launched without training and allows to leverage on the social interaction by the users of the data products. Value needs to be "regular", not one-time. Data needs to be operationalized, not seen as just another project.
Data quality is a never ending journey. Measurement is absolutely essential.
Flipping the odds
Helena Hörnebrant, Director Operation and Transformation - Sweden Scania
Covid meant an operational transformation inside a digital transformation inside a business landscape in full transformation. AI offers exciting opportunities but it requires to operate in ecosystems. It also means that organizations may need to change their ownership models which leads to huge transformations of the financial model and financial operations. Organizations cannot grow anymore due to cost control. Yet, demand is growing. So the mindset needs to flip. What is needed? Integrated strategy, leadership commitment, high caliber talents, agile governance mindset, effective monitoring of progress and business led modular technology.
During a transformation, you need to be aware of the typical Understanding the Change Curve (shock, denial, frustration, low energy, experiment, decision, integration) and manage it actively. Understand where people are on the curve and remedy with the right actions at the right stage.
Modern Data Strategy SubTrack
My notes do not reflect the full program, as I can only attend selected sessions.
Driving data intelligence with data megatrends
Matt Turner, Alation, Director Industry Strategy and Partner Marketing Strategy
Data management leaves a number of questions on the table, such as How to find information?, Can it be used? Should it be used?
Quote from Shelly Palmer: Data rich or data poor, companies with the greatest ability to turn data into action are going to win.
We should go from data driven decision making to data inspired decision making, by Jayant Dhamne.
With regard to data megatrends, most innovations are incremental, adding to the stack, with data as an afterthought. Additional hardware, software, services, models, etc. is just adding up and up and up, but we never clean up. The inclination might be to centralize. Centralization allows for better discovery, control, monitoring and normalization. However, we don't do it for many reasons. And those reasons are valid, so centralization will not bring salvation.
Today's megatrends are "Data Mesh", "Modern Data Stack" and "Data Fabric".
The central theme is to embrace decentralization. What needs to be centralize is governance, training, metadata, collaboration tools, search & discovery and the reconciliation of semantics. Other aspects should be decentrally managed.
Data culture involves Data Search, Data Governance, Data Literacy. Data Literacy is essential because there is no surplus of data skills in the market. So you need to develop these skills yourself as well. Governance can be distributed by making it just a natural part of everything we do, like "putting on a seatbelt". Data Literacy helps to institutionalize otherwise quite theoretical concepts.
Living with Data Strategy at Helen
Mikko Muurinen, Head of Data & AI, Helen Oy
There is a move from very central production of energy to multiple sources, such as sea water pumps, data centers, houses, geothermal sources, industry, bio plants, etc. Both the energy production as well as consumption has become more complex. Both problems are fundamentally "data related". Consumer behavior, energy production and energy storage needs to be aligned. On top of that, there is a pressure to become carbon neutral. A lot of mechanisms are similar to financial markets, e.g. futures, hedging, etc. Some use cases include: employee performance and retention, smoke detection, predictive maintenance, customer segmentation, customer churn, and many more.
The first data strategy was created 2 years ago. There was not a clear roadmap at that point. The data strategy was made as easy as possible, so that the success of the strategy did not rely on anybody reading it. Key areas in the data strategy are: data and analytics to all, better customer experience, data driven innovation, optimization and management of energy system, predictability and stability of business.
So how does Ellen execute its data strategy? The areas to concentrate on are: methods, skills, technology, all three supporting a data culture. A Data & AI unit supports the entire organization with a centralized competency. A Data Academy offers opportunities for employees to learn the basics of data literacy. A data community fosters active networking and communication. Ellen went towards modern and scalable cloud solutions. Self service capabilities were rolled out to different functions. Ellen went towards more agile tribes to foster co-creation. Finally, a solid partner network was established.
The development steps of the strategy include: an organization in tribes and teams, evolved technologies on cloud first, building of capabilities through communication and data academy, data governance with a focus on data quality and data catalog and responsible data & AI, location intelligence with a GIS platform and external data, self-service with courses and better models for support and finally streaming data with a new event architecture and a new streaming data platform.
The data strategy is being kept alive by incrementing it with different policies, e.g data guidelines for external partners, responsible and ethical use of data & AI, update about location intelligence, a data sharing policy and concluding in a digital strategy.
Bringing your Data Strategy to Life
Karolina Perzon, Head of Governance & Strategy, Telia
A consultant advised to establish a Data Governance function. What was the starting point? Telia was in a reality with a massive data legacy, complex IT source structure, many data quality issues, lack of tool support (paralysis by analysis) and a lack of common processes. Telia wanted to take a pragmatic approach.
The following ambitions were set: provide data self-service four technical users (and only after maturity scale to business users), treat data as an asset but most valuable assets first, have data driven business decisions based on high quality data, data democratization by enabling a common language.
The five key areas of the data strategy are: a technical foundation as the enabler, processes and organization, common language, communication and training and privacy & security (by design).
Sequentially, first a data governance framework was defined, a technical foundation was put in place, the organization was set, a focus was kept on privacy and security and the usage was scaled.
Some key take-aways to conclude: define your own best practices (what to follow and where to diverge, what will work for you), fail fast and adjust (make small mistakes rather than big ones), there is no silver bullet (no one product, process or best practice).
Implementation of a data strategy is a journey.
A modern, business focused data strategy with Collibra leveraging data mesh principles.
Tom Dejonghe, VP Product Operations, Collibra
Mission of Collibra is to bring governed, accessible and trusted data to change things for the good. Current legacy environments of enterprise data warehouses, BI platform and data lakes now move to the concept of the "lake house". Similar issues exist, i.e. centralized and monolithic architectures, tightly coupled pipelines and siloed and hyperspecialized ownership. This creates friction and frustration at the side of the data consumers.
Data Mesh is not a tool, not an application. It's more a principle. It's a decentralized sociotechnical approach in managing and accessing analytical data at scale. More power goes to the lines of business who need to take full ownership. Four core principles are: domain data ownership (organizational structure, flexible operating model), treat data as a product (data catalog, data quality, data lineage), self service data infrastructure platform, federated governance.
The goal of governance is to build trustworthy products that people want to use. They know it's safe to use it, because it is governed.
Data Mesh, Data Governance and The Concept of Data Products
Nicola Askham – The Data Governance Coach
Data Mesh is more than technology, it's about the democratization of data and evolution will be needed to get there.
Data Mesh involves a distributed architecture. Data will be available in multiple places. It requires a change in mindset as it is very much a "people thing". Data belongs to the business. Governance should be federated. No one team in the organization can understand all the data. Centralized data governance cannot work. Therefore, the idea of federated data governance is not that shocking.
The democratization aspect of data leads to the need for data products. Can any data be a data product? Would it be useful for a piece of data to become a data product? The question is: what is a data product? A definition could be: "a data set that is valuable on its own and is shared with users". A more sophisticated set of requirements could be: data products need to be accessible, understandable, discoverable, interoperable and trustworthy.
Standard Data Governance Frameworks don't really work. It is not designed for your organization. It can be a valid source of inspiration. Roles and responsibilities are a key element of data governance. Roles include Data Owners, Data Stewards, Data Producers, Data Consumers, Data Custodians and Data Governance Managers. With the advent of Data Mesh, a new role could be that of the Data Product Owners. And further on, a Data Product Development Team (IT) may be required.
Analytics in operational strategy and transformation to drive decision making in large scale IT operations
Niladri Dutta, Global Director & Head of AI Ideation & Data Strategy, Ericsson AB
Purpose of this presentation is to bring in the thought process on how to transform operations by bringing in data as differentiator to make operations more efficient, effective and smarter.
Typical problems are low data quality, extreme legacy with regard to data models. The industry is under cost pressure, especially on the CIO and CDO to make operations more efficient. Key challenges for telecoms include growing demand for end to end digitization, complex business and operational processes, integration of IOT and Cloud to improve existing systems, leveraging new opportunities, optimizing customer engagement.
Transformation in the context of digital transformation often means that you need to plan and assess, design and build and then implement. Here, the emphasis is on operational transformation. For this, advanced data management and then operationalizing it, is required.
The following steps can be taken: understand and document business process, then go to process logic, process rules, critical data elements, data quality monitoring, process performance measurement and potentially adjusting the business model.
There are no magical AI models to "fix the operations".