Notes and insights from the CDAO convention in Frankfurt
4 October 2022
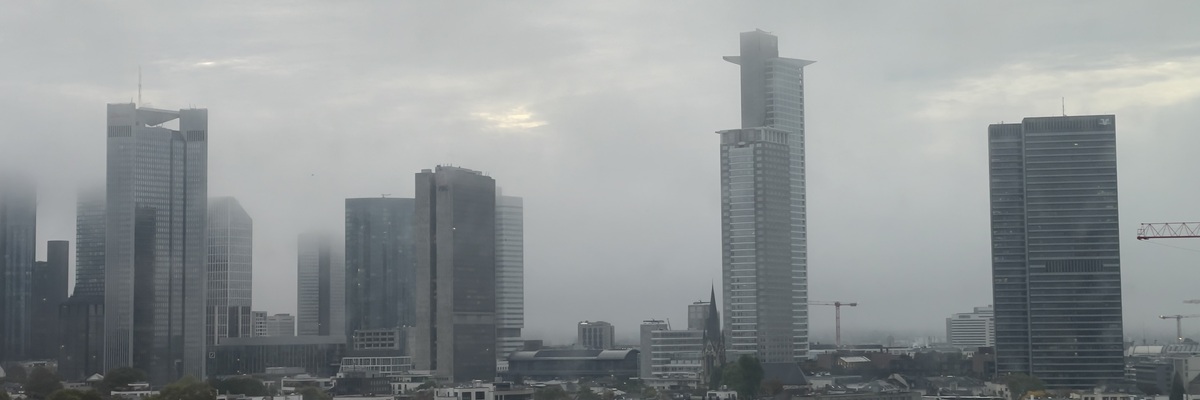
CDAO Germany 2022, Frankfurt
Unless otherwise mentioned, all quotes or positions reflect the positions of the speakers, not of Data Merit.
Implementing a holistic operating model for data
Chris Daniels, Chief Data Officer, Deutsche Bank, Corporate Bank
A holistic approach to an operating model for data consists of three distinct fields, i.e. product building (execution towards the outcomes that are important), data management (governance, oversight to build trust) and caring for platforms and tools (underlying tech that supports the outcomes you want).
The reason of existence for data management and data governance is to create trust in the data that will end up in the data products.
The journey started with a focus on data products with a first use case. The initial reason to have a CDO was to have a product strategist for data. After having defined a first use case, in 10 weeks a prototype was built and the system was in production in 10 months, for a cost that was significantly lower than what was foreseen as a complete platform revamp. From that use case, new use cases were defined. Experience shows, that when things are under your control, it is easier to achieve results. Later, the role went up to "divisional level" and scaled agile was introduced. QAP's, a rather large number of tribes, planning based on fixed capacity, plan publicly, express interdependencies... CDO started leading "data services tribe" which includes data core platform, operations, pipelines, data records management, ownership, data science team as center of expertise,... Scaled agile is experienced as "hugely rewarding". It is easier to course correct and be successful.
It is very hard to predict what will happen in 2-3 years. Data sovereignty is growing as an issue. It will be important to design "for change", to be flexible in dealing with the upcoming unknown.
With regard to the discussion about centralized data teams or not, the result seems to be that both are needed. Decentrally, people need to own their data, feel the pain, get reward for their innovativeness with new products. However, you cannot allow multiple satellite teams to figure out new evolutions, to explore, all separately. The debate should not be binary.
Concluding with a set of lessons learned.
1. An agile operation model is absolutely critical.
2. Share mistakes and failures so that they are not being repeated.
3. Don't start with platforms if the purpose is not clear and clearly accepted.
4. Do not delay getting started (data quality perfection, standards, platforms,...).
5. Nothing beats production data, the quicker you get to using real data, the faster you get results and have reliable moves to production.
6. Data is not the outcome, data governance is not an outcome in itself, it is only a way to make data reliable.
7. The challenges are immense, fostering a positive mindset is very important.
Panel discussion, Liberty + Data + Democracy = Genuine Business Transformation
Helena Schwenk, Vice President, Chief Data & Analytics Office Exasol
Michael Haszprunar, Chief Data Scientist, My BMW App, BMW Group
Robin Jose, Chief Data & Analytics Officer, Wefox
What is data democratization? "Doing the right things with data"? "Just opening up data"? "Giving more and more users access and tools to data"? "Moving organizations further in using data in decision making"? The path towards data democratization may not be obvious. Challenges include data governance, data literacy, having technology stack to enable properly, corporate culture,...?
From a consumer point of view, this is all about empowerment, so that people can do stuff themselves. It is about opening up and tearing down walls. Reliance on central team to decrease. (personal note: does anyone have an objective measure for the perceived inefficiency or efficiency of central teams versus decentral teams?).
The question for democratization is: "where to start?". Identify key strength areas, e.g. customer experience, understanding customers for underwriting process, focus on trust and be aware that every employee can be a stakeholder. Stakeholder segmentation is essential. Passive stakeholders just want their data to be delivered to them, not everybody wants to be an analyst. The more active stakeholders want to have a standard basic set of data but they want a well controlled environment to qualify their data, e.g. by changing dimension values. Then there are the "tourists", i.e. they all want the same thing. The "explorers" ask the question: "hey what else do you have?". These are the hardest to satisfy.
In terms of barriers, language is very important. We need to be aware that "data people" use a lot of jargon, which can be a barrier for regular business people. Co-creation with business people of metrics and dashboards is essential. Data democratization allows data science teams to focus more on "the cool stuff". Standard KPI monitoring should be democratized. Furthermore, there are technical and cultural challenges. In a smaller organization (e.g. 200 people), you can still do things manually. For larger organizations, automation becomes essential for data democratization scaling. Data can be looked at in a defensive way, i.e. don't give access by default. The offensive way is to look only at use cases that generate money, regardless sometimes of data risks. A data officer needs to marry the offensive with the defensive aspects.
In terms of decentralization versus centralization, the hunger cannot be satisfied once it is there. It also allows that data scientists focus on the hardest stuff. It is not about economies of scale, it is about the cost of not making good decisions at the right time. It is also about "understanding the business", which is more difficult centrally.
One of the best a central team can do is to provide consistent standards to act as guardians but also as enablers.
Prioritization between various demand across functions to deliver data should be based on "the need of the many" over "the need of the few". Getting data to as many people as possible should take priority over depth of information for the happy few. A capacity for a "fast track" should also be reserved.
Biggest lessons learned:
1. get the basic platform right or you will not be able to scale
2. data quality becomes huge challenge once data is democratized, e.g. how do you signal individual data pollution?
How An Analytics For All Approach Accelerates Digital Transformation
David Sweenor, Senior Director, Product Marketing, Alteryx
Newvantage Partners survey 2022 says that 92% percent of organizations continue to invest heavily in data and AI but only 19% feel that they have truly established a data culture.
Tom Davenport's "Competing on Analytics" refers to the five stages of Analytic Maturity, with most companies scoring 2.2.
Typically, to answer one analytical question, 6 inputs are needed, generating 7 outputs and requiring 4-7 tools (source: IDC, State of Analytics and Data Science, 2019).
In many organizations, there is a growing divide between experts and non-experts. There is a limited number of projects that the experts can deliver.
90% of processes run on spreadsheets. (source: IDC, 4 ways to unlock transformative business outcomes from analytics investments, 2022)
We need to move to analytics for all. 1. Make analytics easy, 2. Cover everything, 3. Be everywhere and 4. Enable everyone. (personal note: easier said than done and does this not generate the potential of chaotic data consumption).
Best practices for analytics programs include:
1. Executive support, analytics is not the strategy but it enables the strategy.
2. Centre of Excellence and Enablement to train-the-trainer.
3. Snackable training and Education sessions.
4. Set up a community.
5. Use analytics maturity as a guide, organizationally as well as individually.
6. Hackathons & Demo Days.
7. Reward and Recognize.
Panel Discussion: Building a state of the art cloud based technology stack for data analytics
Ulrich Hohmann, Sales Director, Central Europe, Denodo
Mario Vrdoljak, Head of Data & Analytics Consumer Health, Bayer
Susan Wegner, VP Artificial Intelligence & Data Analytics, Lufthansa Industry Solutions
Anna Louise Schroeder, Head of Data Office, AXA Germany
What are the reasons to go to a cloud stack?
1. Scaling
2. Flexibility
3. Concurrent use and self-service
4. Decommissioning of legacy
5. Being future proof for compliance
Some technologies that were mentioned are: Microsoft Azure Data Lake Storage, Microsoft Azure Machine Learning, Rapid Miner, Apache Kafka, Azure Synapse, Airflow, Openshift, Cassandra, DataHub an AWS, Snowflake, Amazon Sagemaker, Microsoft Azure PowerBI, Data Virtualisation with Denodo, Databricks.
You need to solve the efficiency concern that after a while, many use cases reuse similar data assets. For instance, 30% of all use-cases use customer data.
Some pitfalls that were mentioned:
1. Friction to access meta-data should be low.
2. You are often not as cloud-ready as you think you are.
3. Integrate security and data protection from the very beginning.
4. Do not migrate data in a big-bang approach, think about the use-case.
5. Have more than one migration partner.
6. Make sure to have people that are dedicated, not doing this as a side-project.
All companies in the panel, operate in a multi-cloud reality, with Microsoft Azure, Amazon AWS and Google Cloud. You need to be able to get data from multiple cloud providers but it is recommended to provide the central analytics products from one single cloud provider. Avoidance of duplication of functionalities needs to be balanced with addressing the users' needs.
Technology stacks will continue to evolve. Special attention is to be put on managing the meta-data. Another attention point is monitoring the accuracy of models. ESG requirements will start to put pressure on how data is stored, how modelling is done, how the run-footprint of IT is minimized. Natural language processing is evolving extremely fast and existing use-cases should be recalibrated against the fast evolving capabilities. Quantum computing should be kept on the monitor.
Make sure to check that all IP that you put into a cloud platform can be extracted again. Multi-vendor strategies are key.
Exploring AI Through Intelligent Capacity Management in Deutsche Bahn
Thomas Thiele, Chief Expert AI, Deutsche Bahn
Michael Drass, AI Development Manager, Deutsche Bahn
Deutsche Bahn's main concern - in the context of this presentation - is punctuality. 50.000 construction sites generate a huge challenge to manage traffic and ensure punctuality. Intelligent capacity management should support the connection between the planning view of the trains during operations and the ex-post reality. That should enable better operational decisions. The cycle between planning and ex-post evaluation needs to be accelerated.
The planning view of infrastructure works is analyzed proactively and traffic bottlenecks are projected 30 weeks into the future. Bottlenecks have a very big impact on punctuality. Therefore, proactive avoidance of bottlenecks is being performed.
Another factor is potential delay during an individual train journey. Train run data and infrastructure data is used to predict how traffic will evolve in the next 30 minutes. The AI system will provide a recommendation, e.g. to switch one train before the other.
It is investigated whether there are regional clusters of events of rail operations, based on data coming from tachographs and the automated train steering system.
A bottom-up approach, where operators embrace the new system first, ensures top level commitment. This works better than a top-down approach where an AI initiative is imposed on the operators.
Overcoming Your Organisation’s Data Quality Breaking Points
Michael Haszprunar, Chief Data Scientist – My BMW App, BMW Group
Quality is "not a state", data quality is a "spectrum". For BMW, there are a lot of moving parts with lots of potential issues. Issues can be visible to everyone or only by experts after a deep-dive. Data that looks ok, is the most dangerous. You need to look below the surface.
You need to break your own KPI definition. Is your KPI definition is less than an A4 description of text, then it is probably not complete. Think about describing, identifying, examining, specifying, predicting and then cheating your KPI's. KPI calculation should happen in only one place. Code related to the generation of customer (app) analytics should be standardized and not reinvented for every app functionality.
Important KPI's require 4 eyes handling. Identify what is normal (this is quite hard) and avoid manual checks. Create the ability to deep dive. Find external verification sources. Verify what other people think they derive from your data.
If a quality issue pops up, don't panic, communicate proactively. Maintain a data issue historic record. Check explicitly for side effects. Learn and adapt. If you suspect bad data quality, take the access away because no data is better than bad data.
The best quality data is useless when you work with bad KPIs. Be aware of people and the data they create. Build trust by talking about failures and measures against them.
Panel Discussion Data Strategy – Monetizing Your Data
Hidden Treasures – Making Data the Most Indispensable Aspect of Corporate Environments
Thomas Ruske, Data Governance Advisor, Informatica
Gareth Farr, Head of Data and Analytics, HypoVereinsbank – UniCredit *NEW*
Alireza Dorfard, Head of Market Data + Services, Deutsche Boerse
Jo Coutuer, Former Chief Data Officer – Member of the Executive Committee, BNP Paribas Fortis
Max Siebert, CEO, Replique
No notes available